CEA Lunch and Learn: Wei Long
Race Room (201), LBC
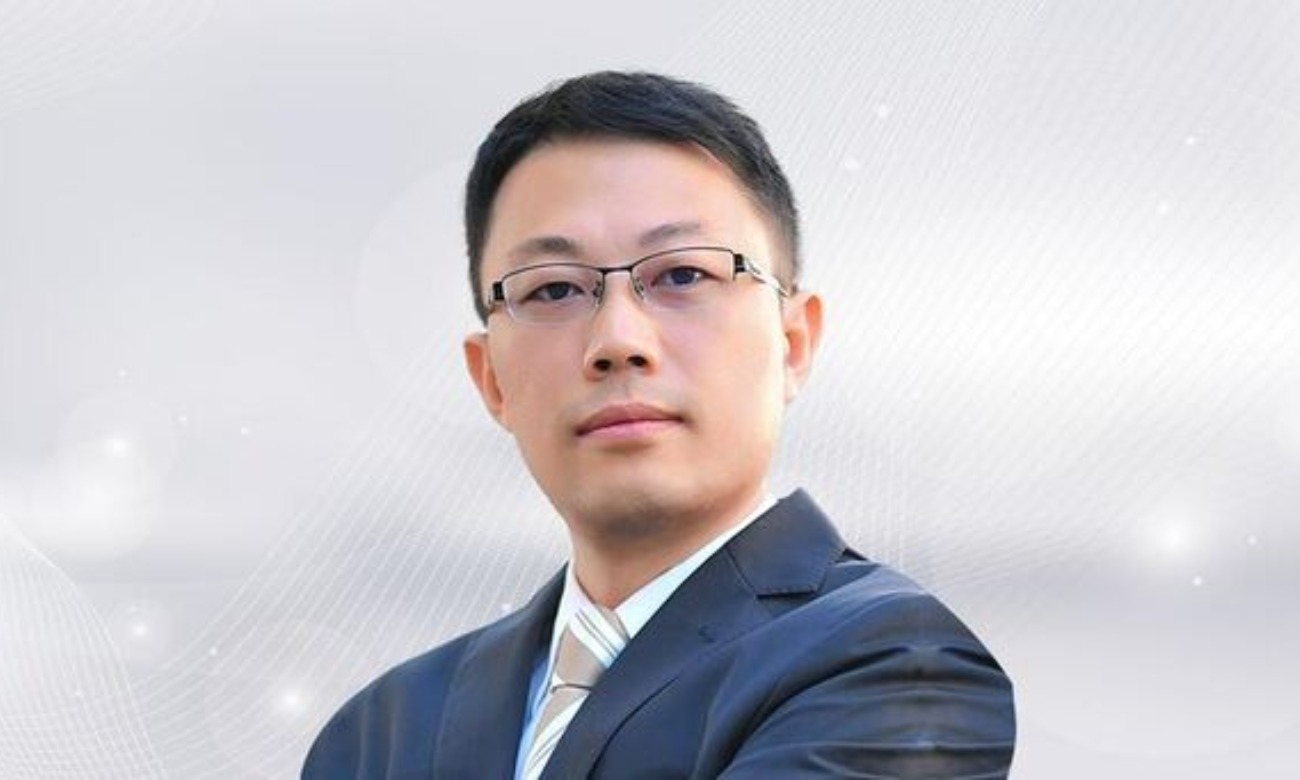
The Center for Community-Engaged Artificial Intelligence and Connolly Alexander Institute for Data Science invite you to the next "Lunch and Learn" seminar exploring the impacts of AI and Data Science on society.
Speaker: Wei Long, Associate Professor of Economics, Department of Liberal Arts
Tulane University is committed to providing universal access to all our events. Please contact Meg Keenan at mkeenan@tulane.edu or 504-862-8381 for accessibility accommodations.